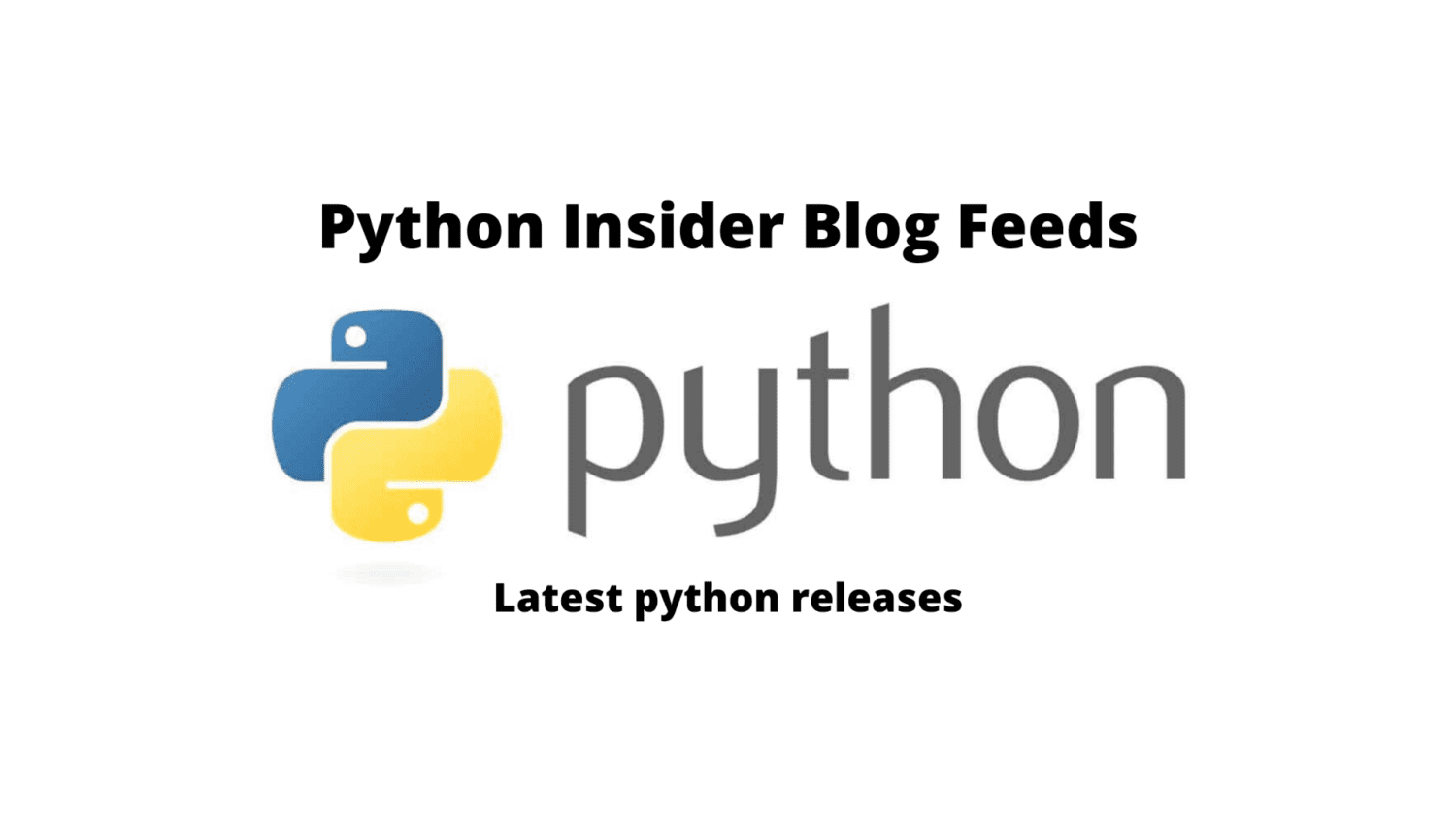
Python 3.12.0 alpha 6 released
I’m pleased to announce the release of Python 3.12 alpha 6.
https://www.python.org/downloads/release/python-3120a6/
Major new features of the 3.12 series, compared to 3.11
- Even more improved error messages. More exceptions potentially caused by typos now make suggestions to the user.
- Support for the Linux perf profiler to report Python function names in traces.
- The deprecated wstr and wstr_length members of the C implementation of unicode objects were removed, per PEP 623.
- In the unittest module, a number of long deprecated methods and classes were removed. (They had been deprecated since Python 3.1 or 3.2).
- The deprecated smtpd and distutils modules have been removed (see PEP 594 and PEP 632). The setuptools package (installed by default in virtualenvs and many other places) continues to provide the distutils module.
- A number of other old, broken and deprecated functions, classes and methods have been removed.
- Invalid backslash escape sequences in strings now warn with SyntaxWarning instead of DeprecationWarning, making them more visible. (They will become syntax errors in the future.)
- The internal representation of integers has changed in preparation for performance enhancements. (This should not affect most users as it is an internal detail, but it may cause problems for Cython-generated code.)
- (Hey, fellow core developer, if a feature you find important is missing from this list, let Thomas know.)
More resources
- Online Documentation
- PEP 693, the 3.12 Release Schedule
- Report bugs at https://github.com/python/cpython/issues.
- Help fund Python and its community.
And now for something completely different
Let me not to the marriage of true mindsAdmit impediments. Love is not loveWhich alters when it alteration finds,Or bends with the remover to remove:O, no! it is an ever-fixed mark,That looks on tempests and is never shaken;It is the star to every wandering bark,Whose worth’s unknown, although his height be taken.Love’s not Time’s fool, though rosy lips and cheeksWithin his bending sickle’s compass come;Love alters not with his brief hours and weeks,But bears it out even to the edge of doom.If this be error, and upon me prov’d,I never writ, nor no man ever lov’d.
Enjoy the new releases
Responses