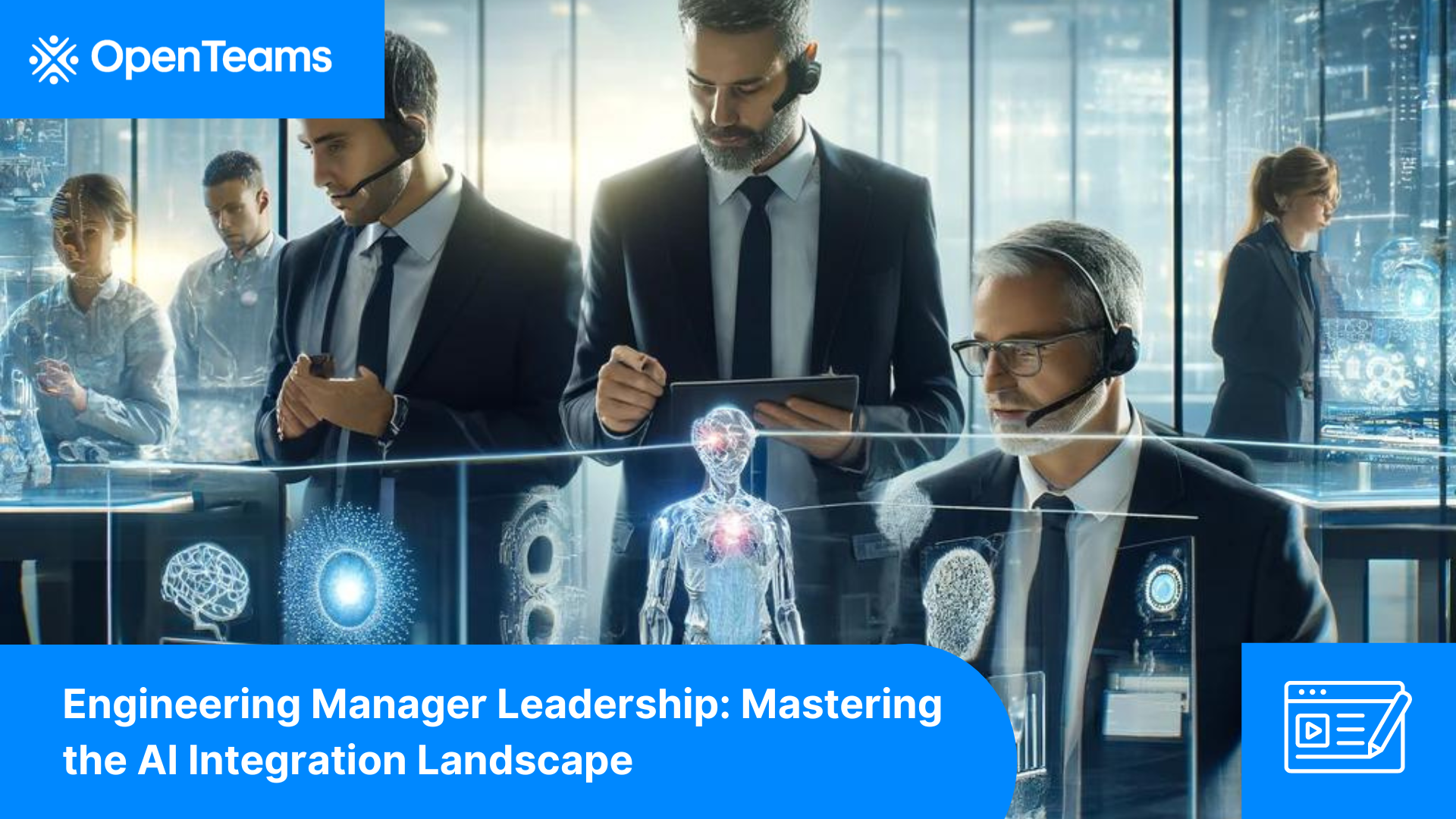
The role of engineering managers is pivotal in the current technological epoch, especially when it comes to integrating Artificial Intelligence (AI) into organizational infrastructures. This complex transition promises to enhance operational efficiencies and unlock innovative potential but comes laden with challenges that demand strategic planning, a profound understanding of AI technologies, and their implications on existing systems. Drawing on the insightful dialogue between Steven Tedjamulia and James Smith, the CTO of Cartec IQ, this article delves deeper into the nuances of AI integration for engineering managers, with a particular focus on large language models (LLMs), API utilization, data management, scalability, and ensuring cost-effectiveness.
Discover deeper insights and strategies by joining our upcoming AI integration webinar.
Introduction: The AI Integration Imperative
In the rapidly evolving tech landscape, AI stands out as a transformative force, enhancing decision-making processes, operational efficiency, and customer experiences. The journey of James Smith from a curious innovator to the CTO of Cartec IQ exemplifies the strategic and comprehensive approach required for successful AI integration. This process is not just a technical challenge but a strategic shift demanding a holistic view.
Key Takeaways:
- Highlighting AI’s strategic importance in innovation and operational efficiency.
- The necessity of assessing organizational readiness for AI integration.
- Strategies for transitioning from traditional systems to AI-enhanced architectures.
- The importance of API flexibility, meticulous data management, scalability considerations, and cost management.
Gain a deeper understanding of these key takeaways at our exclusive webinar on AI integration.
I. Setting the Stage for AI Integration
Embarking on AI integration starts with a critical evaluation of existing systems against the backdrop of organizational objectives, ensuring that AI serves as a strategic enhancement rather than just a technological addition.
A. Assessing Organizational and Technical Readiness
Understanding the current technological infrastructure and its readiness to embrace AI is crucial. This involves a comprehensive review of existing systems, software, and hardware capabilities to identify potential gaps or areas needing upgrades or complete overhauls. It’s about aligning AI initiatives with business goals, ensuring that AI integration delivers tangible benefits and aligns with the long-term vision of the organization.
B. Aligning AI Initiatives with Business Goals
The integration of AI should be a strategic decision, driven by clear business objectives. Whether it’s to improve customer satisfaction through personalized experiences, streamline operations for enhanced efficiency, or innovate product offerings, the ultimate goal of AI integration should be to contribute to the overarching objectives of the organization. This alignment ensures that AI initiatives receive the necessary support and resources, and that their impact is meaningful and measurable.
Discover how to align AI initiatives with business goals in our upcoming webinar.
II. Navigating the Transition: From Monolithic to AI-Enhanced Systems
Moving from traditional, monolithic architectures to more dynamic, AI-ready systems involves several critical considerations, particularly around APIs and data management.
A. The Critical Role of APIs in AI Integration
APIs stand as the cornerstone for integrating AI functionalities into existing systems. They offer a layer of flexibility and adaptability, essential for incorporating AI technologies without disrupting the core architecture of the system. An API-first approach allows for the seamless addition of AI features, facilitating easy updates and scalability. Moreover, APIs enable the integration of AI services from various providers, offering the flexibility to choose the best-in-class AI functionalities and ensuring that systems can evolve with the rapid pace of AI advancements.
B. Data Management: Foundation of AI Efficacy
At the heart of effective AI integration lies robust data management. AI technologies, especially large language models, thrive on vast datasets to deliver accurate and insightful outputs. Optimizing the data infrastructure involves not just the efficient storage and management of data but also ensuring its quality, security, and accessibility. Strategic data planning is crucial for AI’s success, as the quality and structure of data directly influence the AI model’s effectiveness. This means establishing clear protocols for data collection, processing, and storage, ensuring data integrity and privacy, and enabling easy access to data for AI applications.
Learn about the foundational role of data management in AI at our next webinar.
III. Ensuring Scalability and Managing Costs in AI Projects
For engineering managers, scalability and cost are paramount in the AI integration process. These factors determine the feasibility and sustainability of AI projects.
A. Scalability Considerations in AI Deployment
Scalability is crucial, especially for organizations aiming for global reach. Engineering managers must design AI infrastructures that can scale not just vertically but also horizontally, accommodating increased loads without compromising performance. This involves leveraging cloud technologies, microservices architectures, and serverless computing to ensure that AI functionalities can grow with the organization’s needs. The ability to scale efficiently supports international deployments, ensuring consistent AI performance across different regions and accommodating varying data privacy laws and regulations.
B. Balancing Cost with Innovation
Cost considerations are integral to AI integration strategies. While AI offers significant potential for innovation and efficiency gains, its implementation can be costly, particularly in terms of data storage, processing, and the computational power required for training AI models. Exploring cost-effective architectures, such as microservices and serverless computing, can help manage these expenses. Additionally, a thorough cost-benefit analysis can aid in communicating the value of AI investments to stakeholders, emphasizing long-term gains over initial expenditure. Effective cost management ensures that AI projects are not just innovative but also sustainable and scalable.
Join us in our upcoming webinar to explore how to balance cost with innovation in AI projects.
Conclusion: Embracing AI as a Strategic Imperative
For engineering managers, the journey towards AI integration is marked by strategic decisions, technical challenges, and operational considerations. This process demands more than technical expertise; it requires a vision for the future, a deep understanding of AI’s potential, and the strategic alignment of technology with business objectives. By focusing on critical areas such as API flexibility, robust data management, scalability, and cost-efficiency, engineering managers can navigate their organizations through the complexities of AI integration, positioning them for success in a rapidly evolving technological landscape.
Embark on Your AI Journey:
- Learn from our AI integration case studies
- Connect with our experts for personalized advice
- Enhance your team’s skills with our AI courses
- Join our community at upcoming AI events
The insights from Tedjamulia and Smith illuminate the path forward, offering a detailed roadmap for engineering managers aiming to leverage AI’s transformative potential. By addressing each aspect of the integration process with careful consideration and strategic planning, engineering managers can ensure their organizations are well-positioned to capitalize on the opportunities AI presents.
Discover more and deepen your knowledge by registering for our exclusive webinar on AI integration for engineering managers.