
Pioneering the Path to Predictive Healthcare: Longitudinal AI Models Redefine Precision Medicine
Introduction
In the era of precision medicine, artificial intelligence (AI) and machine learning (ML) have emerged as powerful tools to revolutionize healthcare. One area that shows immense promise is the development of longitudinal AI models that take into account an individual’s health trajectory over time. Such models offer a more comprehensive assessment of a person’s health compared to traditional single time-point models. A recent study exploring the combination of body composition imaging and meta-data in predicting 10-year mortality revealed intriguing insights that can shape the future of predictive healthcare.
The Power of Longitudinal Models
Traditional AI models in healthcare have excelled at analyzing single time-point data, offering valuable insights for diagnosis and treatment. However, longitudinal models, which integrate data from multiple time-points, open up new possibilities in predictive healthcare. These models recognize the dynamic nature of human health, capturing changes and time dependencies that provide a more holistic understanding of an individual’s well-being. By mapping these changes to specific health outcomes, longitudinal models have the potential to predict health trajectories more accurately, thereby facilitating proactive interventions and personalized care.
Challenges in Longitudinal Model Development
Developing longitudinal AI models has historically encountered challenges, primarily due to the complexities of curating and managing longitudinal medical data. Unlike single time-point data, longitudinal data involves tracking a patient’s health information over extended periods, often across multiple healthcare institutions. This demands meticulous organization and management, making the curation process time-consuming and costly. Moreover, conducting long-term studies poses issues with patient retention and data collection, which can introduce biases and affect the reliability of the models.
The Study: Predicting 10-Year Mortality
To evaluate the potential of longitudinal models in predicting all-cause mortality, researchers utilized data from the Health, Aging, and Body Composition (Health ABC) study. This longitudinal study followed over 3000 older adults for up to 16 years, providing a rich dataset that included body composition imaging and meta-data such as age, BMI, grip strength, and walking speed.
The researchers developed three types of models: single record models using meta-data, single record models using body composition imaging, and combined sequential models using both meta-data and imaging. The latter proved to be the most effective, demonstrating that imaging contains valuable information beyond what meta-data can capture alone.
The Power of Imaging and Metadata Combination
The study’s findings showed that both meta-data and imaging are crucial in predicting 10-year mortality. However, the combination of body composition imaging with meta-data resulted in the best performance in both single record and sequential models. This suggests that imaging provides additional predictive insights that are not fully captured by meta-data, making it a valuable asset in developing accurate longitudinal health models.
Longitudinal Models Outperform Single Record Models
The research also highlighted the superiority of longitudinal models over single record models, regardless of the data type used. Longitudinal models showcased better performance across all modeling approaches, emphasizing the importance of modeling changes and time dependencies in health data. The Integrated Discrimination Improvement (IDI) analysis further substantiated the benefits of combining imaging and metadata, showing an improvement of approximately 5.8% in model performance.
Clinical Implications and the Road Ahead
The implications of these findings in predictive healthcare are vast. Longitudinal models can revolutionize care delivery by providing precise, personalized predictions about a patient’s health trajectory. By incorporating both metadata and imaging, AI/ML models can offer a more nuanced and accurate picture of a patient’s health, allowing for proactive interventions and improved care outcomes. The study’s insights into variables closely related to high 10-year mortality probability can also guide clinicians in addressing modifiable factors to enhance longevity and healthspan.
Although the development of longitudinal models presents challenges, researchers are working to overcome these obstacles and make the approach more scalable. As the infrastructure and institutional support for efficient data curation improve, longitudinal AI/ML models are poised to transform the landscape of predictive healthcare, bringing us closer to the vision of precision medicine.
References
Final DXA-nation
Lambert T Leong, PhD
Deep learning predicts all-cause mortality from longitudinal total-body DXA imaging
Yannik Glaser, John Shepherd, Lambert Leong, Thomas Wolfgruber, Li-Yung Lui, Peter Sadowski & Steven R. Cummings
Related Articles
Unlock the power of open source for your business today
OpenTeams provides businesses with access to a team of experienced open source professionals who can help them unlock the power of open source technologies, delivering customized solutions tailored to their specific needs and goals. Get in touch with us today to learn how we can help you leverage open source to achieve your business objectives.
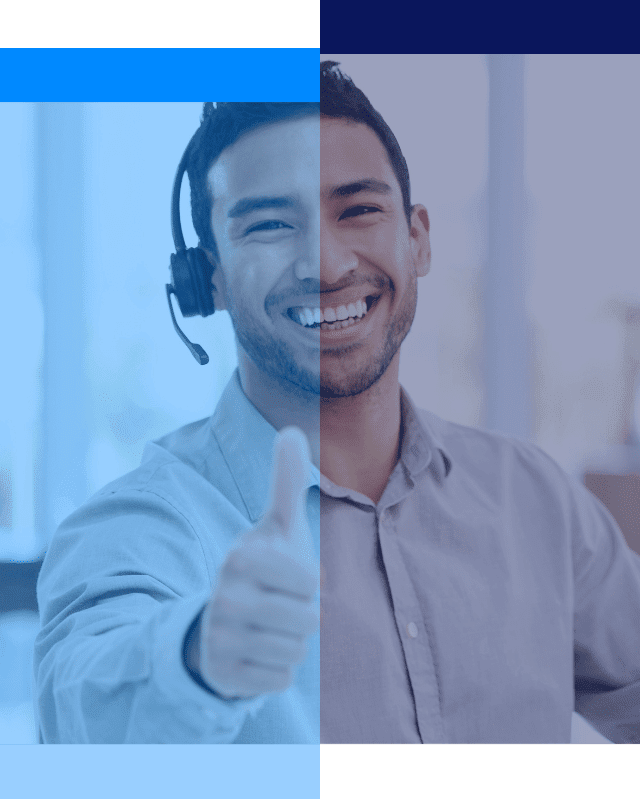